Deep learning is a subset of machine learning based on artificial neural networks. These neural networks mimic how the human brain learns, enabling them to learn from data without being explicitly programmed.
As deep learning continues to evolve, we can expect even more impressive advancements in the field. Deep learning will play a key role in improving our understanding of natural language processing and image recognition. Additionally, it will help us create more accurate models for predicting outcomes and prescribing actions. To peer into what the future holds, we’ll look at five deep learning trends we can expect to continue in 2022.
1. Improvements for Specific Tasks Such As Natural Language Processing
Deep learning algorithms have significantly improved natural language processing (NLP). Specifically, it has helped us better understand the meaning of words and phrases, as well as the context in which they are used. As a result, we have better machine translation and information retrieval.
Deep learning will continue to play a key role in improving NLP. We’re particularly looking forward to seeing how machines progress in their understanding of text sentiment. This improvement will especially benefit business applications like customer service and marketing. For example, deep learning is refining chatbot and digital assistant technology — helping people resolve issues and better routing them before they need to talk to a rep.
2. New Deep Learning Architectures and Algorithms
Deep belief networks (DBNs) were created to solve some of the challenges of deep learning, like slow performance and difficulty with massive data sets. DBNs are composed of several layers of neural networks that do not communicate horizontally or within their own layer. This structure makes it more efficient in its usage of neural network layers, speeding up complex tasks and improving performance when working with large data sets. Furthermore, DBNs are flexible and able to employ both supervised and unsupervised learning.
3. Leveraging Deep Learning Model Scale Up to Improve Accuracy
Deep learning model scale up is a term used to describe the process of increasing the size and/or complexity of a deep learning model. Scaling up can improve the accuracy and speed at which the deep learning model can learn.
There are two main ways to scale up deep learning models:
-
- Add more layers. Adding more layers or nodes can improve accuracy by helping the model learn more complex patterns and process more data. However, users must be strategic when adding layers, as too little and too many can impact your algorithm’s performance.
-
- Increase its size. Increasing the size of the deep learning model can improve its speed since the model can parallelize the learning process more effectively than a smaller deep learning model.
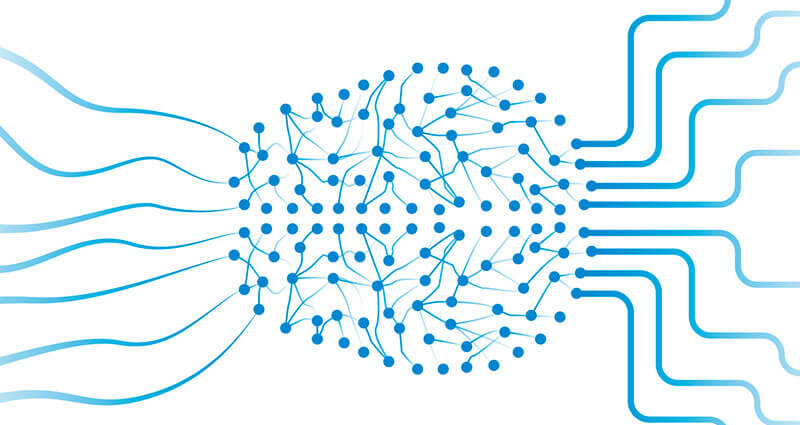
4. Evolution from Predictive Modeling to Prescriptive Action
Deep learning has also made predictive modeling more accurate. In the past, predictive models were often based on shallow machine learning algorithms that struggled to learn from high-dimensional data. Nowadays, deep learning algorithms can learn from data with many features, making them more accurate.
As deep learning progresses, it will help us create predictive models that are more accurate and easier to interpret. Moreover, deep learning will better understand the cause-and-effect relationships in data, making it possible to prescribe actions rather than simply predict outcomes.
5. Greater Accessibility for Non-Data Engineers
Deep learning algorithms are often seen as complex and difficult to understand. But this is starting to change as deep learning becomes more accessible. Many tools and services now make it easy to develop deep learning models, even for those with limited experience. Consider DALL·E 2 from OpenAI as an example. DALL·E 2 allows users to easily generate unique artwork using AI by inputting natural language descriptions.
Since algorithms are becoming more general, advancements in unsupervised learning are also making deep learning more accessible. Users can now reuse models for different use cases, limiting the need for specialized expertise.
Deep Learning Is Changing How We Use AI and Machine Learning
Deep learning is profoundly impacting how we use AI and machine learning in the real world. And in the future, it will continue to change how businesses use AI and its prevalence in their applications. Therefore, to prepare your business to handle more deep learning applications, it’s important to ensure that your company’s hardware provides adequate performance.
Equus Compute Solutions helps companies design, deploy, and maintain high-performance computing solutions. We can help you determine the right level of computing infrastructure for your business today and in the future. If you want to talk more about how you can build out your deep learning infrastructure, let’s talk.