[et_pb_section admin_label=”section”] [et_pb_row admin_label=”row”] [et_pb_column type=”4_4″][et_pb_text admin_label=”Text”]
Data is a constant for businesses. However, the type, quantity, and size make all the difference when extracting insights. Even small organizations can have thousands, if not millions, of data points. And it’s not feasible to analyze data at this scale manually.
Machine learning makes data analysis at scale feasible — allowing teams to leverage computing hardware to do the heavy lifting. However, even within the realm of machine learning, you must decide which types of learning, algorithms, and hardware are needed to help you extract trustworthy results. We’ll consider different machine learning methods and why you might choose one over the other.
Match Your Learning Method to Your Data
Supervised and unsupervised learning are both subsets of machine learning. But, while related, they work very differently.
Supervised learning uses labeled data to train algorithms to predict outcomes, while unsupervised learning analyzes unlabeled data to find patterns and group similar data together.
Imagine you want to train a machine learning algorithm to predict prices in your industry for the following year. To produce reliable results, you’d need to feed it pricing data from several years and data on factors that impact price, current demand, etc. You’d also have to feed it labeled data (data tagged with one or more labels), and once the algorithm is sufficiently trained, you can use it to get an accurate view of next year’s prices.
On the other hand, unsupervised learning doesn’t need labeled data. However, it wouldn’t be ideal for the previous example since unsupervised learning isn’t used to make predictions. Instead, you can use it to understand the relationship between data.
For example, if you have millions of data points on your customers, labeling it all would be time-consuming and costly. Instead, you could use unsupervised learning to analyze data in real time, looking for patterns that you could then analyze for valuable insights. Companies like Pinterest, Facebook, and Twitter have already been doing this for years — leveraging machine learning to learn about user preferences and tailoring their feeds to be more engaging.
Align Your Machine Learning Approach to Your Goals
Supervised vs. unsupervised learning isn’t a case of one method being better, but rather different. Just like a hammer and a screwdriver help you accomplish different tasks, these machine-learning methods need to be aligned with your goals to be effective. Accuracy requires that you start by analyzing your company’s goals. To help, we’ve listed some ideal applications and shortcomings for each.
Ideal Applications
- Supervised learning is best suited for projects where you want to predict outcomes or need a high level of accuracy. This type of learning is great for tasks like predicting behavior and trends and identifying images.
- Unsupervised learning is best when you want to learn from large data sets and/or unlabeled data. These projects tend to produce unexpected insights that may provide a competitive edge.
Shortcomings
- Supervised learning requires labeled data which can be time-consuming and labor-intensive to create. Labeling the necessary data also requires specialized talent.
- Unsupervised learning can produce inaccurate results if you don’t have an expert to validate the variables. Additionally, this type of learning requires a more robust computing infrastructure.
Both approaches to machine learning have their strengths and weaknesses. Therefore, choosing the right one requires you to align its strengths with your project’s needs. Consider what type of data you have available (labeled vs. unlabeled), what kind of problem you’re trying to solve (well-defined vs. open-ended), and the talent you have access to (experts for labeling, data scientists, etc.).
However, sometimes you run into a situation where you have tons of data and need a high level of accuracy. In these cases, a hybrid approach tends to work best.
Get the Best of Both Worlds With Semi-Supervised Learning
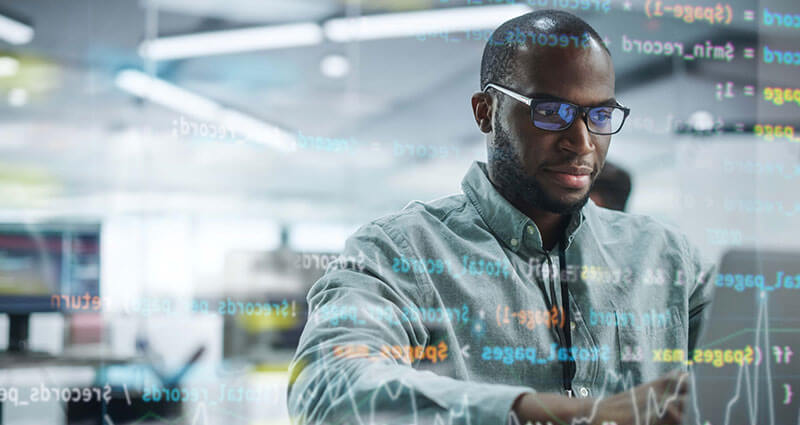
In many real-world applications, obtaining labeled data is challenging, while unlabeled data is abundant. Semi-supervised learning allows businesses to use their abundant data without incurring impractical labeling costs.
Semi-supervised learning combines a small amount of labeled data with large amounts of unlabeled data during the training process. It leverages the information from both types to improve the model’s performance which benefits businesses in three key ways:
- Cost-effective data labeling. Semi-supervised learning reduces the need for extensive labeled data and the corresponding expertise, enabling businesses to save money and allocate resources more efficiently.
- Improved model performance. Semi-supervised learning can lead to better model performance than supervised learning models trained on a limited amount of labeled data. As a result, you’ll get more accurate predictions and better decision-making.
- Scalability. Semi-supervised learning enables businesses to scale their machine learning efforts more easily by leveraging larger datasets without incurring high labeling costs.
Semi-Supervised Learning Enhances Your Team’s Capabilities
Semi-supervised learning gives your team access to different methods and techniques that improve efficiency, accuracy, and speed. To provide you with an idea of the capabilities you gain, we’ve outlined a few below:
- Self-training. In self-training, a supervised model is initially trained using the available labeled data. The model is then used to predict labels for the unlabeled data. The most confident predictions are returned to the training set with their predicted labels, and the model is retrained. This process is iteratively repeated to improve the model’s performance.
- Co-training. Co-training involves training two or more models on different views (i.e., subsets of features) of the labeled data. Each model then predicts labels for the unlabeled data, and the most confident predictions are shared between the models. The models are retrained with the updated labeled data, and the process continues iteratively to improve their performance.
- Multi-view learning. Multi-view learning is similar to co-training but uses a single model with multiple views of the data. The model learns from the labeled data and leverages the relationships between different views of the unlabeled data to improve its performance.
- Label propagation and label spreading. Based on graph theory, these methods construct a graph where data points are nodes, and edges represent the similarity between data points. The labeled data points are used to initialize the graph with known labels. The labels are then propagated or spread throughout the graph based on the similarity between neighboring nodes, effectively transferring label information from labeled to unlabeled data points.
- Generative models. Generative models, such as Variational Autoencoders (VAEs) and Generative Adversarial Networks (GANs), can be used for semi-supervised learning. They learn a joint probability distribution over the input data and the labels, enabling them to analyze the relationship between different variables and make predictions. By leveraging both labeled and unlabeled data, these models deepen their relationship analysis, improving classification performance.
Semi-supervised learning methods work by exploiting the structure and information present in both labeled and unlabeled data to improve model performance. They are particularly useful in situations where obtaining labeled data is expensive, time-consuming, or challenging.
Preparing Your Organization for Machine Learning
Supervised, unsupervised, and semi-supervised learning can be invaluable tools for organizations leveraging data. These machine-learning methods power everything from speech recognition and forecasting market fluctuations to product recommendations and market segmentation. As organizational data grows, machine learning tools become more critical to a business’s ability to analyze and interpret data in real time — since it becomes a symbolic paperweight without an efficient way to analyze data.
Regardless of the machine learning methods you choose to employ, it’s crucial that your computing infrastructure can handle the load. Equus Compute Solutions has been helping organizations design, deploy, and manage HPC infrastructure for over three decades. Together we can develop hardware solutions that fit your business’s unique needs and are ready to scale. Contact us to learn more.
[/et_pb_text][/et_pb_column] [/et_pb_row] [/et_pb_section]